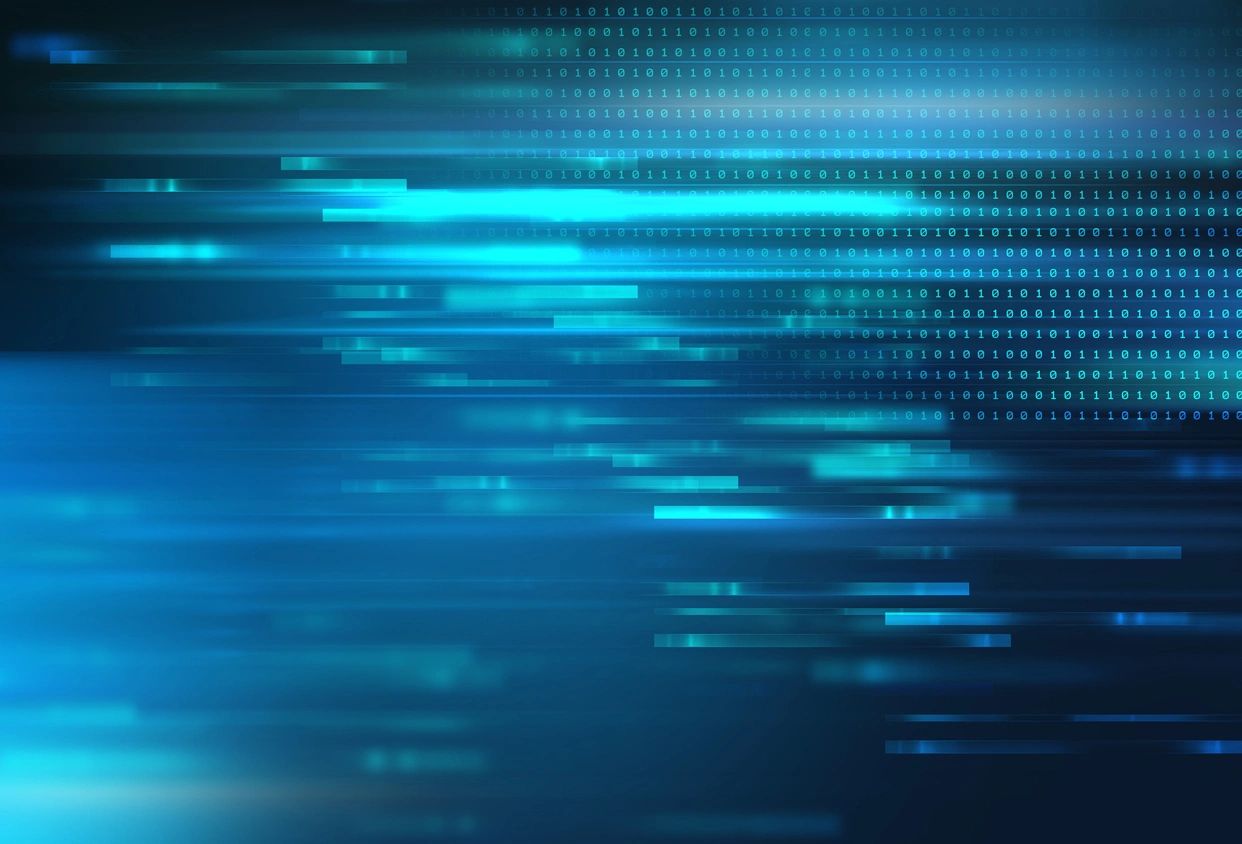
By Muqaddas Mehmood
“Big Data” grows bigger every year, and today’s enterprise leaders not only need to manage larger volumes of data, but they critically need to generate insights from their existing data. Businesses need to stop merely collecting data points and start connecting them. In other words, the relationship between data points matter almost more than the individual points themselves. To leverage those data relationships, your organization needs a database technology that stores relationship information as a first-class entity. That technology is a graph database.
While traditional relational databases have served the industry well in the past in enabling service and process models that tread upon these complexities, in most deployments they still demand significant overhead and expert levels of administration to adapt to change. Relational databases require cumbersome indexing when faced with non-hierarchic relationships that are becoming more common in complex IT ecosystems with partners and/or suppliers and service providers, as well as more dynamic infrastructures associated with cloud and agile.
Unlike relational databases, graph databases are designed to store interconnected data that’s not purely hierarchic, make it easier to make sense of that data by not forcing intermediate indexing at every turn, and also making it easier to evolve models of real-world infrastructures, business services, social relationships, or business behaviors that are both fluid and multi-dimensional.
Current State of Master Data Management:
The world of master data is changing. Data architects and application developers are swapping their relational databases with graph databases to store their master data. This switch enables them to use a data store optimized to discover new insights in existing data, provide a 360-degree view of master data and answer questions about data relationships in real time.
Your Master Data Management (MDM) program likely uses the same database technology as your transactional application: a mature, highly-tuned, relational database (RDBMS). You excel at relational databases because you have many years of experience working with them and most of your data live there, so it makes sense to keep master data there. Traditionally, MDM has included Customer, Product, Accounts, Vendor, Partners and any other highly shareable data in an enterprise.
Master Data, by definition, is highly shared. This tends to cost business agility in a way that ripples throughout the organization. Our architectures struggle on getting data to fit a single definition of the truth, something most of us realize is not a feasible solution in the long run.
The Future of Master Data Management:
MDM programs that attempt to retain data in a single location physically continue to wrestle with the realities of modern Information Technology. Most enterprise organizations use vendor applications: customer relationship management (CRM) systems, work management systems, accounts payable, accounts receivable, the point-of-sale systems, etc. Due to this approach, it’s not always feasible to move all Master Data to a single location. Even with a CRM system in place, we typically end up with customer information maintained in several systems. The same goes for product and accounting data as well.
The most successful programs will not strive to find a single physical location for all data, but will provide the standards, tools, and services necessary to provide a consistent vision of enterprise data. There will be data we can store in one place, using the technologies that best fit its data story. Data will also likely be found in multiple physical systems due to the increasing use of packaged applications as well for performance and geographically-distributed processing needs. Once we understand our environment, we can architect solutions that build upon those needs.
The future of Master Data Management will derive value from data and its relationships to other data. MDM will be about supplying consistent, meaningful views of Master Data. In many cases, we will be able to unify data into one location, especially to optimize for query performance and data fit. Graph databases offer exactly that type of data/performance fit, as we will see below. In this paper, we discuss why your master data is a graph and how graph databases like Neo4j are the best technologies for master data.
Today’s enterprises are drowning in “Big Data” – most of which is mission-critical Master Data – and managing its complex relationships can be quite a challenge. Here are some of the most difficult hurdles in MDM that enterprises must face:
• Complex and hierarchical datasets
Master Data such as organizational and product data has deep hierarchies with top-down, lateral, and diagonal connections. Managing such data models with relational database results in complex and unwieldy code that are slow to run, expensive to build, and time-consuming to maintain.
• Real-time query performance
Master Data systems must integrate with and provide data to a host of applications within the enterprise – sometimes in real time. However, traversing a complex and highly interconnected dataset to provide real-time information is a challenge.
• Dynamic structure
Master Data is highly dynamic with constant addition and re-organization of nodes, making it difficult for your developers to design systems that accommodate both current and future requirements.
The best data-driven business decisions aren’t based on stale information silos. Instead, you need real-time Master Data with information about data relationships. Graph databases are built from the ground up to support data relationships. With more efficient modeling and querying, organizing your Master Data in a graph yields relevant answers faster and with more flexibility than ever before.