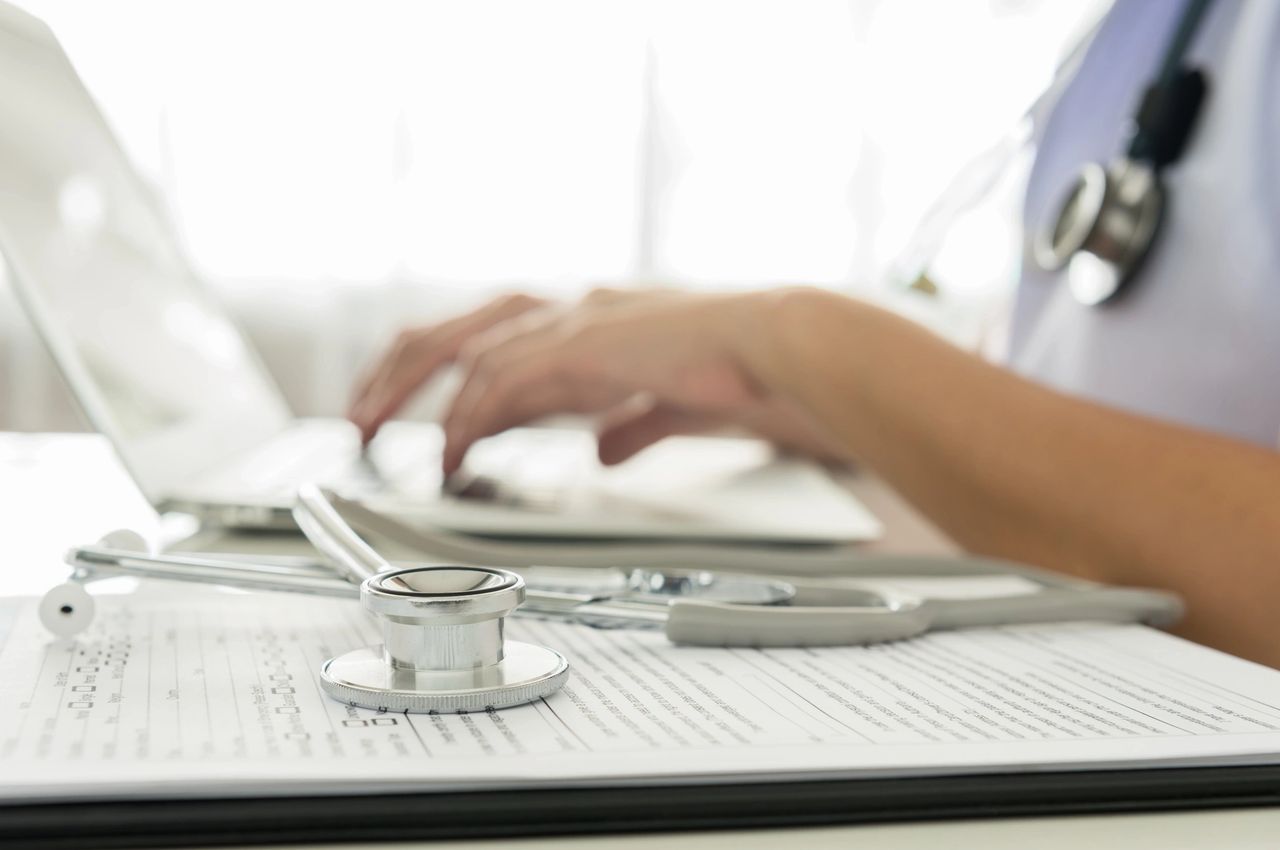
By Mery Ramirez
Why should data integrity, collection, and reporting matter to a health professional? The words “integrity” and “integration” may sound similar, but they have a vastly different meaning, particularly for health professionals. Data integrity means keeping your data clean, useful, and whole. Data integration refers to putting your data together. Both processes are vital for making Big Data work.
The practice of data analysis, collection, and reporting is different from theory. It’s more than just automated processes. It’s a vital piece in the puzzle of data. Any missing piece will lead to data fragmentation. Data integrity is one of the most promising solutions to that issue. In this article, let’s dig deep into the problems of data integrity, collection, and reporting in health care. And how we can help you bring your health data to the forefront.
Data Integrity, Collection, and Reporting: Explained
Imagine going to the dentist for your regular check-up and you’re asked to fill a form. Looking at this form, you see that it’s actually a photocopy of the original. You can barely read the faded printing on the sheet of paper. The lines are broken, there’s a visible cut at the top, and it’s generally in a bad shape.
This photocopy was photocopied from a copy of the original document. The original quality is lost. In short, data integrity degrades over time. Reusing, reproducing, and storing data will affect integrity, collection, and reporting.
That example isn’t different from what happens to digital data.
Imagine an original piece of data as a first-scan document. It’s identical to the original document. Next, imagine you printed this scanned version, photocopied the printed version, and scanned the photocopy again. Then, you snapped a photo of the screen with your phone, you sent the screenshot to a colleague who printed it with a printer running low on ink. At this point, the original document has changed drastically. It’ll lose its integrity. That’s also what happens to health data. And it’s near impossible to restore the document to its original state.
You ask your patients, suppliers, and colleagues to upload data on various devices on conflicting systems. You create copies of this data and store it somewhere else. In the process, you create duplicates and backups using different technologies and change their formats. What’s more, you might migrate to a new system causing more changes to the data.
As you can see, collecting data isn’t easy. And preserving its quality poses a challenge for healthcare facilities.
The Seven Deadly Sins of Health Data
- Entering data without validation
- Uploading different versions of the same dataset
- Importing and exporting data without supervision
- Human negligence
- Creating several backups without updating them
- Isolating data on one system without connection
- Not taking data integrity, collection, and reporting seriously
All these “sins” degrade the quality of your data and make it impossible to use. When you want to ensure consistent care for patients or provide health research assistance, you’ll need high-quality data. When you get two conflicting records for the same patient, which one will you use? Even the slightest difference will make accurate tracking and reporting hard to attain.
Data Integrity, Collection, And Reporting: Solved
What to do when you’re responsible for data integrity, collecting, and reporting in your health care facility or practice? First, you should enforce strict rules and protocols.
At least, conduct regular audits for detecting corrupt data. Have routines, checklists, and processes to meet data integrity, collecting, and reporting requirements. Your system administrator should also be aware of any changes.
Furthermore, do weekly or monthly data validation to ensure your data is up to date. Validation gives you an additional pair of eyes on your data.
In ancient times, when scholars and scribes copied a book, they counted every character in every line to make sure it’s an identical copy of the original. Printing changed that. Today, computer programmers take a few seconds to validate data.
Is Data Analysis the Same as Data Analytics?
First, let’s make the distinction between data analysis and data analytics. Many data companies advertise their data analytics features with little attention to data analysis features. Some use the terms interchangeably and cause more confusion.
Data analytics is a vast discipline with many branches like data collection, storing, organizing, visualization, reporting, and more. Data analytics help in gathering business intelligence based on real situations.
Data analysis is another branch of data analytics that’s only concerned with cleaning and scrubbing the data. Unlike data analytics, data analysis mainly focusses on having clean data.
With that distinction in mind, let’s examine the challenges facing healthcare data analysis.
Challenges of Data Analysis in Healthcare
Data analysis is key to the kingdom of health data. It’s critical to the process of gaining insightful information from your health data. Data analysis is the field of cleaning, refining, and inquiring data to extract useful information. It’s the road to reveal the secrets hidden in the data.
Without data analysis, it’ll be near impossible to put health data into work. Gaining actionable insight from your health data would be like searching for a needle in a haystack.
With machine learning, data analysis is now more sophisticated than before. It’s now possible to collect, clean, and visualize a huge amount of health data. However, there is also a huge gap between data analysis systems.
The challenge of analyzing the data is huge, but understanding the data is another. Machine learning is being used to unlock secrets in health data.
Health data can yield valuable insights, but it is a road full of unique challenges. Here are just a few obstacles:
1. When Health Big Data Is Too Big
A great deal of big data is stored on the servers of healthcare facilities, which poses security, privacy, and scaling problems. Although cloud storage is a promising solution, supporting IT infrastructure is still a challenge.
Hybrid storage systems appear to have filled that gap. However, more storage space has always been required to deal with all that data. It’s crucial to have enough storage space to meet the needs of healthcare facilities.
2. Data Cleaning Is Time Consuming
You can automate the cleaning process to save time. Unfortunately, the data isn’t entirely reliable since you cannot always be sure it’s clean. Storing a huge amount of data without ensuring accuracy is a waste of resources. Uncleaned data is bad data. When you use bad data, it’s impossible to make decisions.
Manual data cleaning isn’t any more error-free. And it’s time-consuming. Luckily, machine learning techniques are evolving fast to meet this challenge.
3. Data Comes in All Shapes, Sizes, And Forms
In the current medical recording system, patients’ data is collected differently. That makes categorizing health records difficult. Using different systems to collect medical records create conflicts that are hard to reconcile. Even though coding systems differ, it can still be difficult to see the big picture.
Universal systems will bridge the gap between clinical analytics, billing, and claims. It’s important to remember that health data is not a universal system.
4. High-Quality Data Is Hard to Collect
Due to the inadequate data collection methods, accurate data is hard to organize. Some patients fail to report their symptoms promptly. They neglect to update their health records as well. As a result, the data quality suffers.
Without accurate reporting, clinical data cannot improve. Your data collection method becomes outdated when it cannot keep up with the real world.
5. Capturing Faulty Data
A technical problem with one of the imaging machines may lead to faulty data, resulting in inaccurate data.
When medical images don’t capture the correct problem, it becomes impossible to use. For example, a distorted x-ray scan will have too much noise. Thus, it’s difficult to identify the real symptoms of the patient. It is impossible to analyze data when small mistakes accumulate.
6. Security and Privacy Threats
In the hacker world, health data is always in demand so it can be sold at the highest price. Medical data is a valuable asset on the black market. Security is a big challenge for data analysis because unethical persons view it as the holy grail of personal data.
Patients don’t feel privacy laws are doing enough to protect their data. A vital part of data analysis is the collection and sharing of health information between facilities. Paradoxically, patients are afraid to share their data because of that fear of getting their data shared with the world.
Conclusion
There’s untapped potential for data analysis in health care. But first, it’ll need to evolve past the obstacles. Healthcare professionals will also need to adapt to these changes. There’s no use in analyzing data if you’re not going to use it effectively.
There are still bigger challenges ahead, but data analysis has come a long way already. Right now, it’s impossible to predict the future, but healthcare will surely undergo a dramatic change in the coming years.
The solutions to these challenges will open the possibility of expanding healthcare.
Are you facing some data analysis challenges? Let’s talk and see if we can come up with a solution.